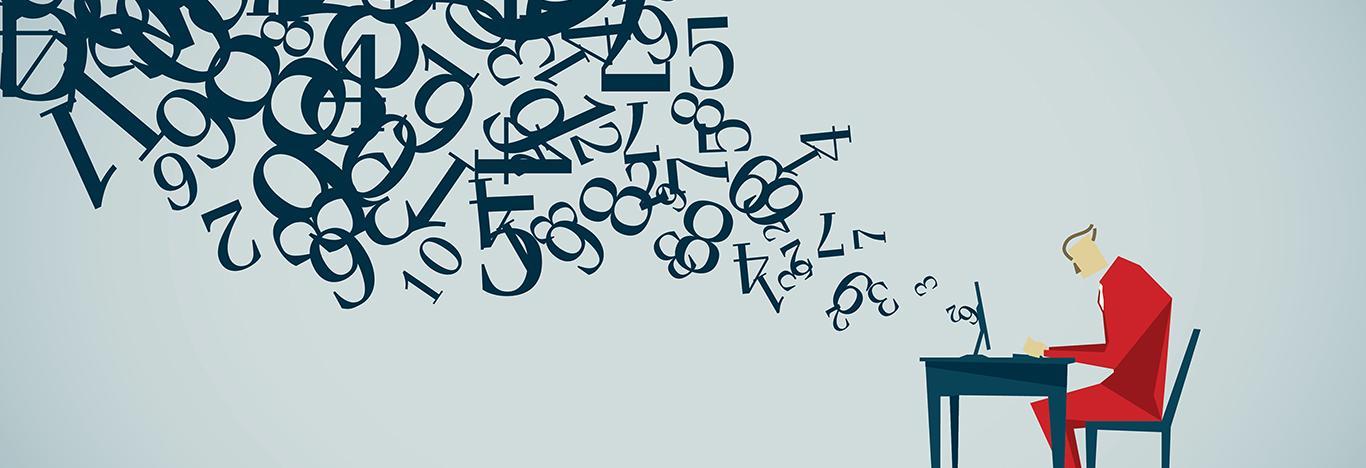
Early successes have buoyed enthusiasm for potential big-data applications in medicine. [1], [2], [3], [4] Algorithms, powered by predictive analytics, can leverage big data to compute prognoses on a minute-by-minute basis. [1], [5] However, designing and using computer programs specifically to provide clinicians with support for clinical decisions in real-time—in the context of scarce medical resources—has ethical implications, which are examined here. Given that computers are free from limitations of human judgment, I argue that, if they are designed specifically to ration care with respect to specified moral and social norms, big data algorithms can promote just allocation of scarce medical resources.
Rationing in Medicine
Rationing is the allocation of a good in the face of scarcity, meaning that some who want and could benefit from a good will not get it. [6] In other words, different parties have an interest in the same scarce resource because each of them will not be as well off without it, yet not everyone can or will receive the scarce resource. Here, I am concerned with the sense of “rationing” whereby goods are equitably distributed, though individuals are not guaranteed health care resources in excess of those allocated. [7] To ration, then, is to determine the equitable distribution of limited benefits within a society, based on guiding principles and societal values. I will describe a system of rationing, which deprives people of medical care they are owed as less just or less fair than it could have been.
There are two distinct types of rationing: macroallocation and microallocation. Decisions within the former, affect statistical or hypothetical lives; those within the latter, affect identified lives. Examples of macroallocation include policies on vaccine distribution during an outbreak, rules of priority on transplant waiting lists, and decisions about apportioning funding for research between common and uncommon diseases. [8], [9] In contrast, microallocation is more context dependent, relying on individual judgment to determine equitable distribution, for example, of beds in an intensive care unit or care during an emergency for existing patients. [10] The relevance of various ethical considerations depends on the type of allocation. [11] This paper will highlight problems posed by human judgment, particularly in the context of microallocation.
Fair Allocation
Just rationing requires a set of guiding principles and social values. [12] Depending on the circumstances, one system of resource allocation may be favored over another. Examples of rationing strategies include those that treat all people equally, favor the worst-off, maximize the total benefits, or promote social usefulness. [9] For example, allocation for liver transplant—determined by recipient MELD-Na (The Model for End-Stage Liver Disease-Sodium), a score of three-month mortality in patients with cirrhosis—prioritizes the sickest patients first. Allocation of organs for kidney transplant, in contrast, gives priority to people who have waited the longest. At times, rationing is designed using a mixed approach. Emergency departments (EDs), for example, allocate medical attention first to urgently ill patients, and then to everyone else on a first-come, first-served basis. [13] This approach achieves some specified balance of distributive justice and social desirability. An acutely ill patient with an urgent and serious disease is the worst-off without medical attention, and potentially stands to benefit greatly from prompt treatment. On the other hand, distributing a good to everyone else according to a queue is widely considered equitable.
While social and moral norms determine how to fairly distribute scarce resources in theory, explicit rules or criteria are required for application in the real world. [14] Consider the following illustrative scenario: Patient A presents to an ED with difficulty speaking and there is concern for acute stroke. Patient B presents with diminished consciousness following a motor vehicle accident and there is concern for intracranial hemorrhage (ICH). Both injuries are potentially serious, and both would demand immediate diagnosis and management to mitigate permanent neurologic sequelae. But whom should the clinician evaluate first? Who would be worse off if not granted priority access to the CT scanner, and who stands to benefit more from being diagnosed first? Without a way to determine who meets the definition of “sickest,” the first recipient of medical attention will be chosen ad hoc, rather than by application of guiding principles and values.
Separate from the normative questions, any system for allocating scarce medical resources should also provide explicit protocols that detail how to distribute goods or services after the initial determination to allocate the goods or services is made. These operational rules or criteria for resource allocation can be adapted and improved to reflect an overarching aim.
The utility of algorithms in rationing is not contingent on the value of a single system of resource allocation, nor the efficacy of some set of rules or criteria; rather, algorithms are adaptable to promote many different ethical goals, depending on one’s overarching aim. Minor tweaks to a system of allocation can, in real time, also better realize an ideal system of rationing.
This argument is therefore agnostic about how to properly articulate the principle of justice in the context of rationing. There are different ways to determine who initially receives priority for resource allocation. While I do not presuppose that any particular system of allocation is the fairest, in what follows, there is an assumption that a system of rationing is more just than failing to ration in practice. In other words, a system of rationing, wherein a person ought to be allocated some resource but does not actually receive it, is less just than a system that provides everyone with the resources they are owed. Instead, I focus on human limitations in actual allocation of resources owed and the extent to which big data algorithms can better realize a fair system of rationing regardless of how justice is defined.
To Err is Human
Clinician judgments and behavior may limit fairness in microallocation. Consider again the example of prioritizing care in the ED for a suspected stroke versus a suspected ICH. In this situation, the guiding rule—most urgent case to the front of the line—is known, but the rule does not state how to apply it in practice. As long as physicians are charged with rationing care, determining who meets which criteria, for subsequent treatment, if any, will necessarily be a matter of individual judgement. [10] Because the rules of allocation are usually not comprehensive, they do not provide anticipatory guidance for every possible circumstance. Algorithms, however, could avoid several constraints in clinician judgment, thereby promoting just microallocation of resources.
The first limitation, in clinician reasoning, is prognostic uncertainty. Would it be different if Patient A had atherosclerotic risk factors instead of atrial fibrillation, or if Patient B had a history of drug use rather than no past medical history? In addition to ambiguity about current medical status, providers may disagree about the prognoses for each patient. A big data algorithm, however, would avoid these concerns. Computers, harnessing predictive analytics, have the potential to explicate prognosis using vast amounts of data. [5] Prognosis can be updated in real time, as more information becomes statistically known. Moreover, while two competing physicians can oversell the severity of an illness in order to advocate for scarce resources on behalf of their patients, algorithms offer uniformity in clinical judgment. Thus, algorithms can better achieve the underlying goals for any given system of rationing.
A second impediment to fair resource allocation is that clinicians may not be psychologically equipped to make or carry out rationing decisions for a number of reasons. First, under the patient-doctor relationship, physicians are obligated to provide the best possible help to the patient, who, as a result of the physician’s rationing decision, might not receive the most or best possible care. This is a professional conflict, which currently all physicians must negotiate. [15] Unlike policy-level macroallocation decisions, a physician may psychologically feel much closer to patients affected by microallocation rationing and, hence, more responsible for their wellbeing. [12] The intuition described as the duty of easy rescue—the feeling that one should help another person when the burdens to oneself are low and the benefits for that individual are great—is widely shared among physicians. [6] In following the instinct to do good for a single patient, physicians inadvertently create an inequitable distribution of goods within a given population as a whole.
"Rather than subvert autonomy or consent, big data algorithms have the potential to engage the public regarding the extent to which scarcity in medicine exists, and how the system is still fair overall."
Computers, on the other hand, are not similarly constrained by conflicts of conscience and cognitive errors. Granted, an algorithm could be programmed to promote certain ways of applying values, such as the duty of easy rescue. However, it could prevent these considerations from affecting resource distribution unless doing so would make rationing more just according to the selected rules or principles governing microallocation. Algorithms could thus apply programmed rules more consistently. Whether Patient A and Patient B are seen by a neurologist or an ED physician, for example, should have no bearing on how resources are allocated. Most conceptions of justice require that cases be treated the same when they share all morally-salient features. [8] Yet, in the real world, who sees the patient may determine microallocation. Avoiding these sorts of psychological susceptibilities by using algorithms could help remove inconsistencies in rationing resources, making the system more fair overall.
Thirdly, it is difficult for clinicians to communicate face-to-face that rationing has affected a patient’s care. [16], [17] It would be understandably challenging to inform a lower-priority patient that she would be better-off receiving available care, but she will not receive that care because of the clinician’s decision to provide it for another person. This barrier to honest communication with patients works against efforts to fully disclose information to patients about their care, and fails to show them due respect.
An algorithm, in contrast, has the advantage of making explicit the need for rationing in medicine, helping align the method of rationing with societal values. Patient-oriented software programs can inform individuals that rationing of specific forms of care will occur. If the patient or family protest about the ED triage decision, a computer could have a preprogrammed set of procedures for appeal. Although appeal is also possible when clinicians currently ration care, most people never find out when providers have rationed care in the first place. [18] A system of rationing, operating under private rules, prevents public discourse about the procedures and unfairly benefits insiders with power. [12] The use of algorithms, on the other hand, makes the rules and policies of rationing explicit, promoting not only their critical evaluation but also allowing society to examine and debate the proper set of values to inform the system of resource allocation. Rather than subvert autonomy or consent, big data algorithms have the potential to engage the public regarding the extent to which scarcity in medicine exists, and how the system is still fair overall.
Caution and Skepticism
Systems of allocation, which overcome problems inherent in human judgment, can promote a more just system of resource allocation. Although big data algorithms hold great promise, they should still be approached with caution and skepticism. Algorithms should not be relied upon to ration medical care until the technology has substantially matured, however, since the argument in favor of using them makes a substantial empirical assumption about the capability of predictive analytic technology to improve fairness in resource allocation. Despite these technical hurdles, a clinical tool that can develop and apply an unbiased and precise system of rationing, agreed upon by all stakeholders, would make great strides in promoting a shared vision of fair allocation in the face of medical scarcity.
J. Bradley Segal, MBE '17, can be reached at BioethicsJournal (at) hms.harvard.edu.
[1] Etheredge, Lynn M. "Rapid learning: A Breakthrough Agenda." Health Affairs 33, no. 7(2014): 1155-1162.
https://doi.org/10.1377/hlthaff.2014.0043.
[2] Weber, Griffin M., Kenneth D. Mandl, and Isaac S. Kohane. "Finding the Missing Link for Big Biomedical Data." JAMA 311, no. 24(2014): 2479-2480. https://doi.org/10.1001/jama.2014.4228.
[3] Esteva, Andre, Brett Kuprel, Roberto A. Novoa, Justin Ko, Susan M. Swetter, Helen M. Blau, and Sebastian Thrun. "Dermatologist-level classification of skin cancer with deep neural networks." Nature 546, no. 7649 (2017): 115-118. https://doi.org/10.1038/nature21056.
[4] Angus, Derek C. "Whether to Intubate During Cardiopulmonary Resuscitation: Conventional Wisdom vs Big Data." JAMA 317, no. 5(2017): 477-478. https://doi.org/10.1001/jama.2016.20626.
[5] Cohen, I.G., Ruben Amarasingham, Anand Shah, Bin Xie, and Bernard Lo. "The Legal and Ethical Concerns that Arise From Using Complex Predictive Analytics in Health Care." Health Affairs 33, no. 7 (2014): 1139-1147.
https://doi.org/10.1377/hlthaff.2014.0048.
[6] Brock, Dan W. "Health Care Resource Prioritization and Rationing: Why is it so difficult?" Social Research vol 76, no. 1 (2007): 125-148. https://www.jstor.org/stable/40971892.
[7] Beauchamp, Tom L. "Childress JF." Principles of Biomedical Ethics. Oxford University Press; 2001.
[8] Emanuel, Ezekiel J. and Alan Wertheimer. "Who Should Get Influenza Vaccine When Not All Can?" Science 312, no. 5775(2006): 854-855. https://doi.org/10.1126/science.1125347.
[9] Persad, Govind, Alan Wertheimer, and Ezekiel J. Emanuel. "Principles for allocation of scarce medical interventions." The Lancet 373, no. 9661 (2009): 423-431. https://doi.org/10.1016/S0140-6736(09)60137-9.
[10] Truog, Robert D., Dan W. Brock, Deborah J. Cook, Marion Danis, John M. Luce, Gordon D. Rubenfeld, and Mitchell M. Levy. "Rationing in the intensive care unit." Critical Care Medicine 34, no. 4 (2006): 958-963. https://doi.org/10.1097/01.ccm.0000206116.10417.d9.
[11] Brock, Dan W. "Separate spheres and indirect benefits." Cost effectiveness and resource allocation 1, no. 4 (2003). https://doi.org/10.1186/1478-7547-1-4.
[12] Caplan, Arthur L. "Organ transplant rationing: a window to the future?" Health Progress 68, no. 5 (1987): 40-45. PMID: 10282293.
[13] Iserson, Kenneth V. and John C. Moskop. "Triage in medicine, part I: concept, history, and types." Annals of Emergency Medicine 49, no. 3 (2007): 275-281. https://doi.org/10.1016/j.annemergmed.2006.05.019.
[14] Gatter Jr., Robert A. and John C. Moskop. "From futility to triage." Journal of Medicine and Philosophy 20, no. 1 (1995): 191-205. https://doi.org/10.1093/jmp/20.2.191.
[15] Brody, Howard and Franklin G. Miller. "The Internal Morality of Medicine: Explication and application to managed care." The Journal of Medicine and Philosophy 23, no. 4 (1998): 384-410. https://doi.org/10.1076/jmep.23.4.384.2572.
[16] Fuchs, Victor R. "The 'Rationing' of Medical Care." The New England Journal of Medicine 311, no. 24(1984): 1572-1573. https://doi.org/10.1056/NEJM198412133112411.
[17] Epstein, Ronald M., David N. Korones, and Timothy E. Quill. "Withholding information from patients—when less is more." The New England Journal of Medicine 362, no. 6 (2010): 380-381. https://doi.org/10.1056/NEJMp0911835.
[18] Coast, Joanna. "The rationing debate. Rationing within the NHS should be explicit. The case against." BMJ (Clinical Research ed). 314, no. 7087 (1997): 1118-1122. https://doi.org/10.1136/bmj.314.7087.1118.
[19] Joyce, James. "Bayes' theorem." The Stanford Encyclopedia of Philosophy (2003) https://plato.stanford.edu/archives/spr2019/entries/bayes-theorem/.
[20] Brehm, Jack W. and Arthur R. Cohen. "Explorations in cognitive dissonance." (1962) https://doi.org/10.1037/11622-000.
[21] Richardson, Robert G. Larrey: Surgeon to Napoleon's Imperial Guard. (Quiller Press, 2000).
[22] Blagg, Christopher R. "Triage: Napoleon to the present day." Journal of Nephrology 17, no. 4 (2004): 629-632. PMID: 15372431.
[23] Lee, Christopher H. "Disaster and mass casualty triage." Virtual Mentor 12, no. 6 (2010): 466. https://doi.org/10.1001/virtualmentor.2010.12.6.cprl1-1006.
[24] United States Department of Defense. "Emergency War Surgery." (Washington, D.C.: The United States Government Printing Office, 1958).
[25] Harris, John. "QALYfying the value of life." Journal of Medical Ethics 13, no. 3(1987): 117-123. https://doi.org/10.1136/jme.13.3.117.